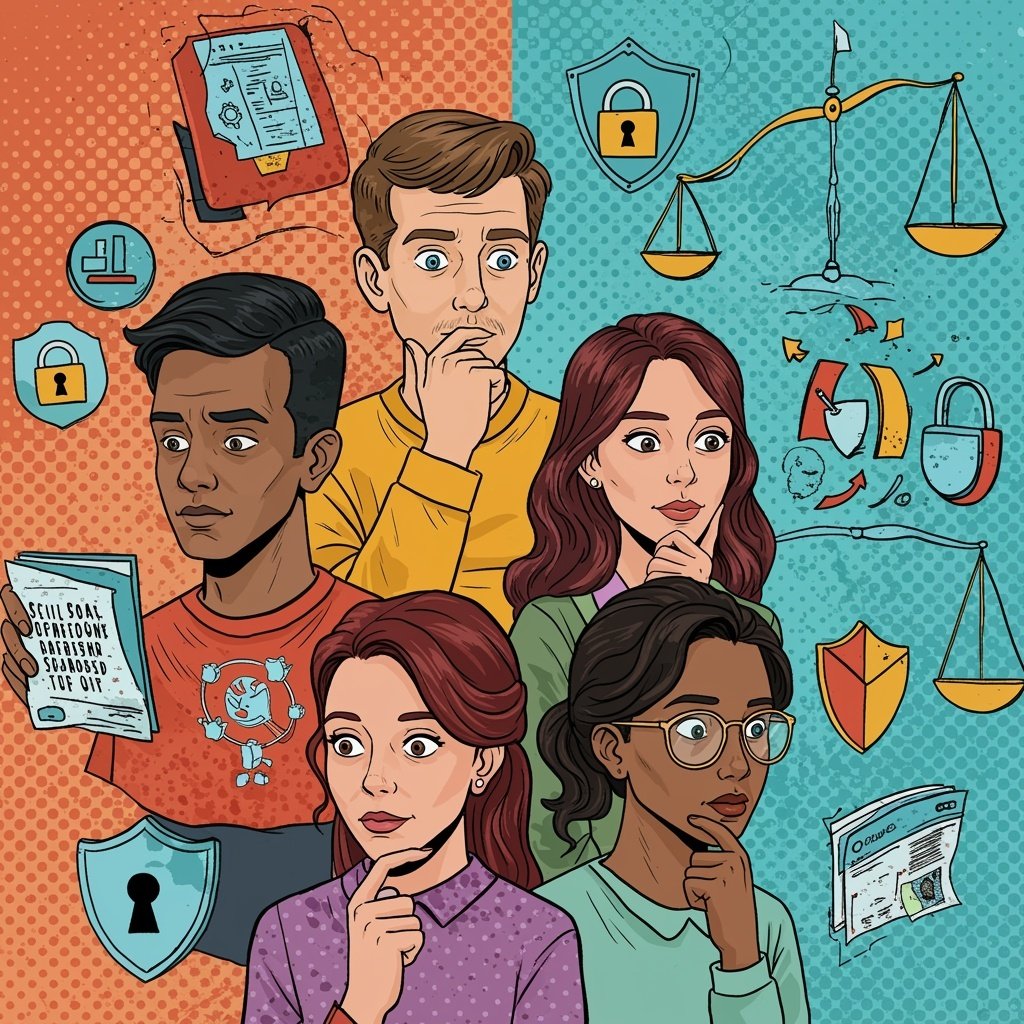
Ethics Is Not “One and Done”
Implementing ethics in Machine Learning is not a one-and-done effort. Organizations must build trustworthy systems that serve their customer’s best interests fairly. Ultimately, ethically designed machine learning models align with compliance and regulations while also avoiding harmful outcomes.
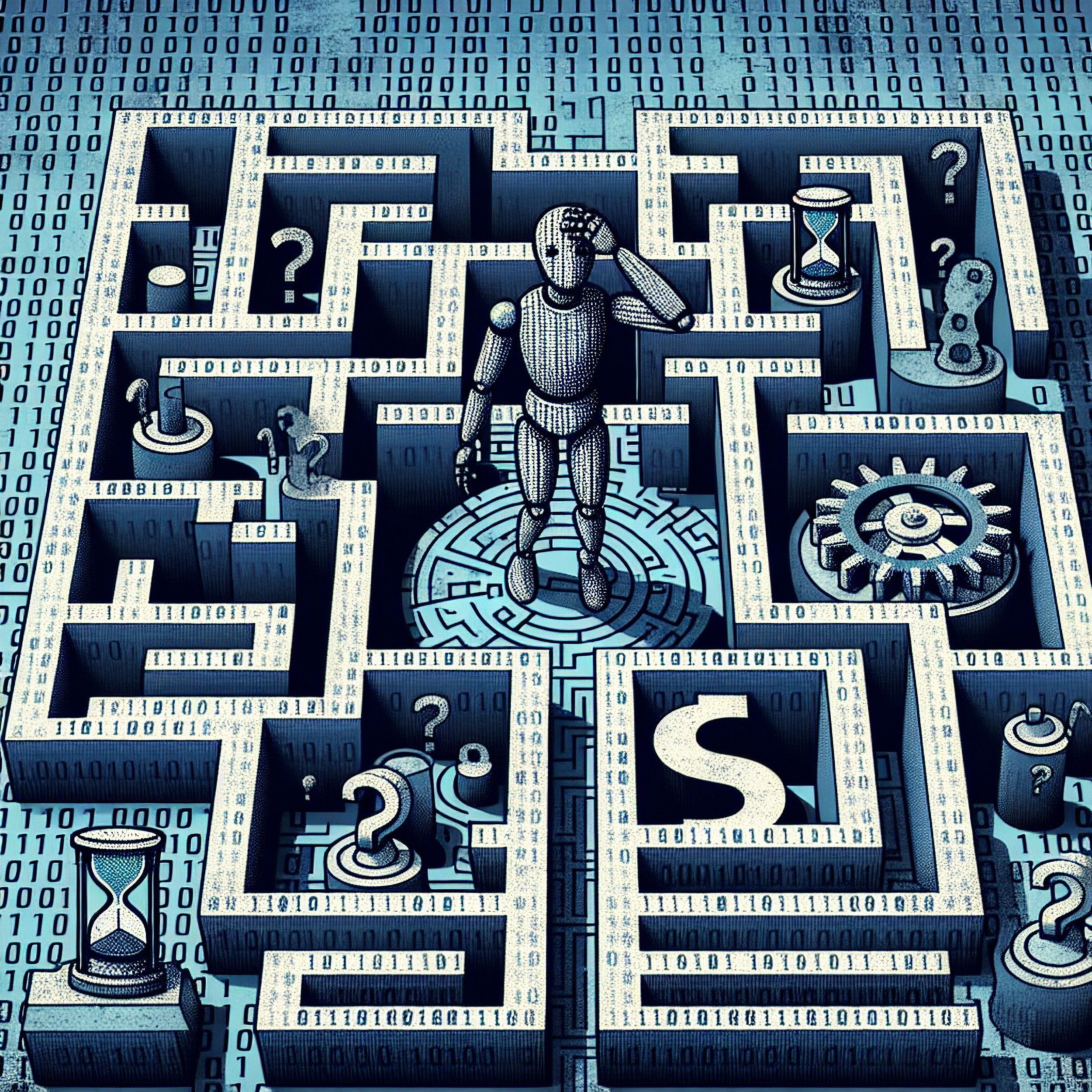
Practical Business Reasons to Resist the Allure of AI
There are many traps along the journey required to leverage AI/ML to generate value for your business. Success relies on aligning AI/ML initiatives with clear business objectives and understanding their true potential.
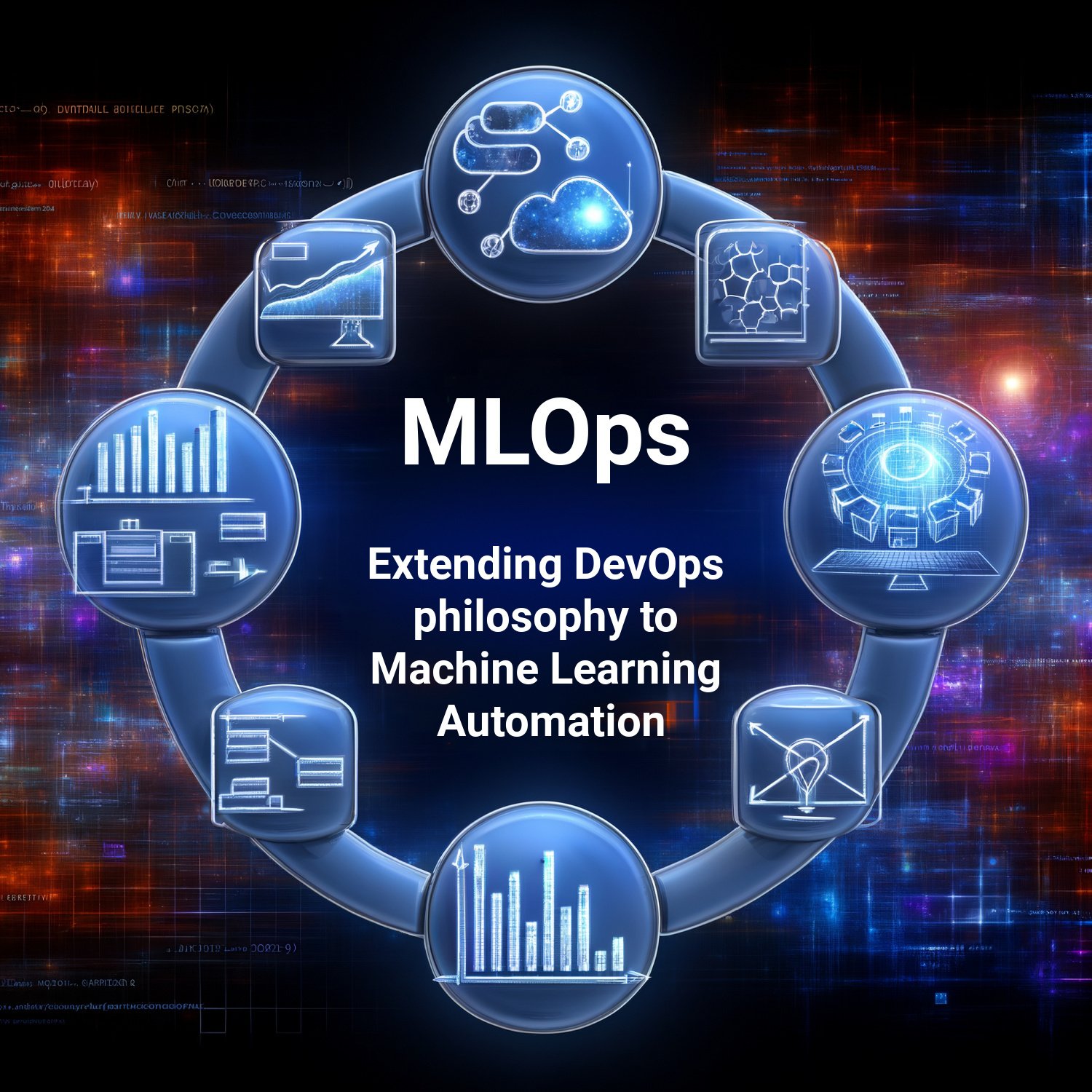
MLOps Automation
MLOps requires specialized knowledge that traditional DevOps teams lack. The challenges related to data quality, consistency, and accessibility demand a different set of skills and tools.
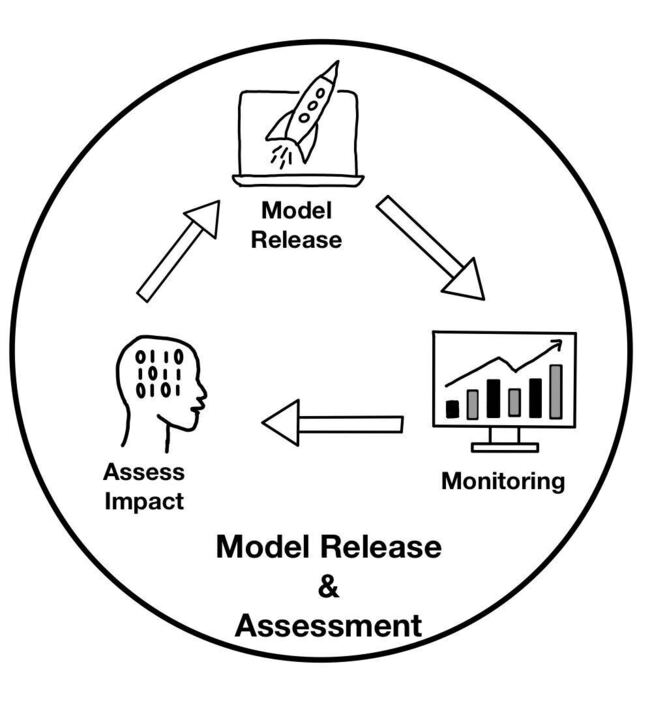
Model Release & Assessment Phase
This 3rd phase of our Data Science Process explores the release of ML models into production and the importance of ongoing monitoring and assessment.
Additionally, it provides a framework for defining "done" and achieving a high-quality model release.